Barriers to Adopting AI in AEC Firms
September 21, 2024
The Architecture, Engineering, and Construction (AEC) industry stands to benefit significantly from the adoption of Artificial Intelligence (AI) technologies, offering the potential to revolutionize design, construction processes, and project management. AI's ability to analyze vast datasets, automate repetitive tasks, and provide predictive insights can lead to enhanced decision-making, improved project efficiency, and cost savings. However, despite these promises, several significant barriers hinder the widespread implementation of AI in this sector. This document explores the challenges, with a focus on data management issues and the strategic steps needed to overcome them.
Vast Amounts of Underutilized Data
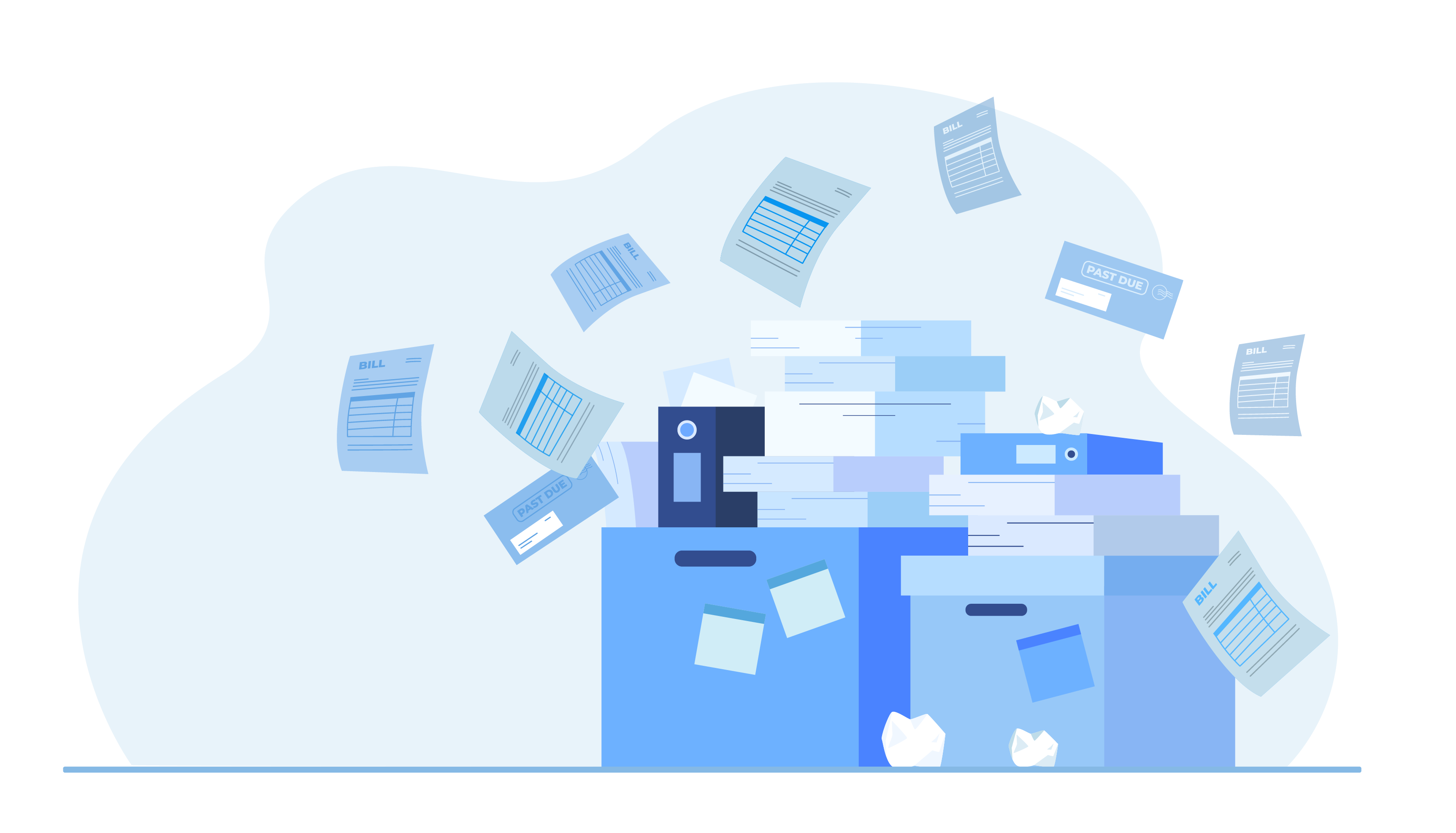
AEC firms have accumulated enormous volumes of data over years of operation. This data includes:
- Past project proposals: Documents detailing client requirements, budgets, timelines, and stakeholder expectations.
- Design documents: Architectural and engineering drawings, including Computer-Aided Design (CAD) files.
- Building Information Modeling (BIM) files: BIM models that contain detailed 3D representations of physical and functional characteristics of buildings.
- Construction plans and specifications: Technical details for construction processes, materials, and methodologies.
This wealth of information, collected over the years, represents a treasure trove of potential knowledge. Unfortunately, much of this data remains underutilized due to several reasons:
- Disorganized storage across various platforms: Data is scattered across different systems such as internal servers, cloud platforms (like SharePoint), and local hard drives, making it difficult to retrieve or use cohesively.
- Lack of standardized filing systems: Different departments and project teams may follow different filing practices, leading to inconsistent data management approaches that complicate access and analysis.
- Employee turnover: As key personnel leave firms, their knowledge and understanding of past projects or data handling practices are lost, creating gaps in context and making it hard to derive insights from historical data.
The failure to leverage this historical data results in inefficiencies, missed opportunities for improving processes, and limits firms' ability to learn from previous projects.
Data Complexity and the Need for Clean Data
.png)
AI systems, particularly those relying on machine learning, thrive on high-quality, well-structured, and relevant data. However, the data accumulated by AEC firms is often far from this ideal state, due to several challenges:
- Inconsistent formatting across different projects and timeframes: Different teams or projects may use varying naming conventions, file formats, and data standards, which creates obstacles for AI tools that require uniformity.
- Mixing of structured and unstructured data: While some data (such as numerical data or schedules) is structured, a significant portion (e.g., reports, emails, meeting notes) is unstructured. Unstructured data is harder to parse, categorize, and use for AI-driven insights.
- Outdated or irrelevant information: Large datasets often contain outdated data or irrelevant information that introduces noise and reduces the efficacy of AI algorithms.
- Lack of standardized metadata and tagging systems: Metadata (data about data) is crucial for categorizing, searching, and organizing datasets, but is often inconsistently applied or completely absent.
The presence of these issues means that a considerable effort is required to clean and prepare data for AI applications. Poor-quality data can significantly degrade the performance of AI algorithms, leading to inaccurate insights, inefficiencies, and poor decision-making. Thus, data preparation becomes a critical bottleneck in the AI adoption process.
![]()
The Challenge of Data Organization and Automation
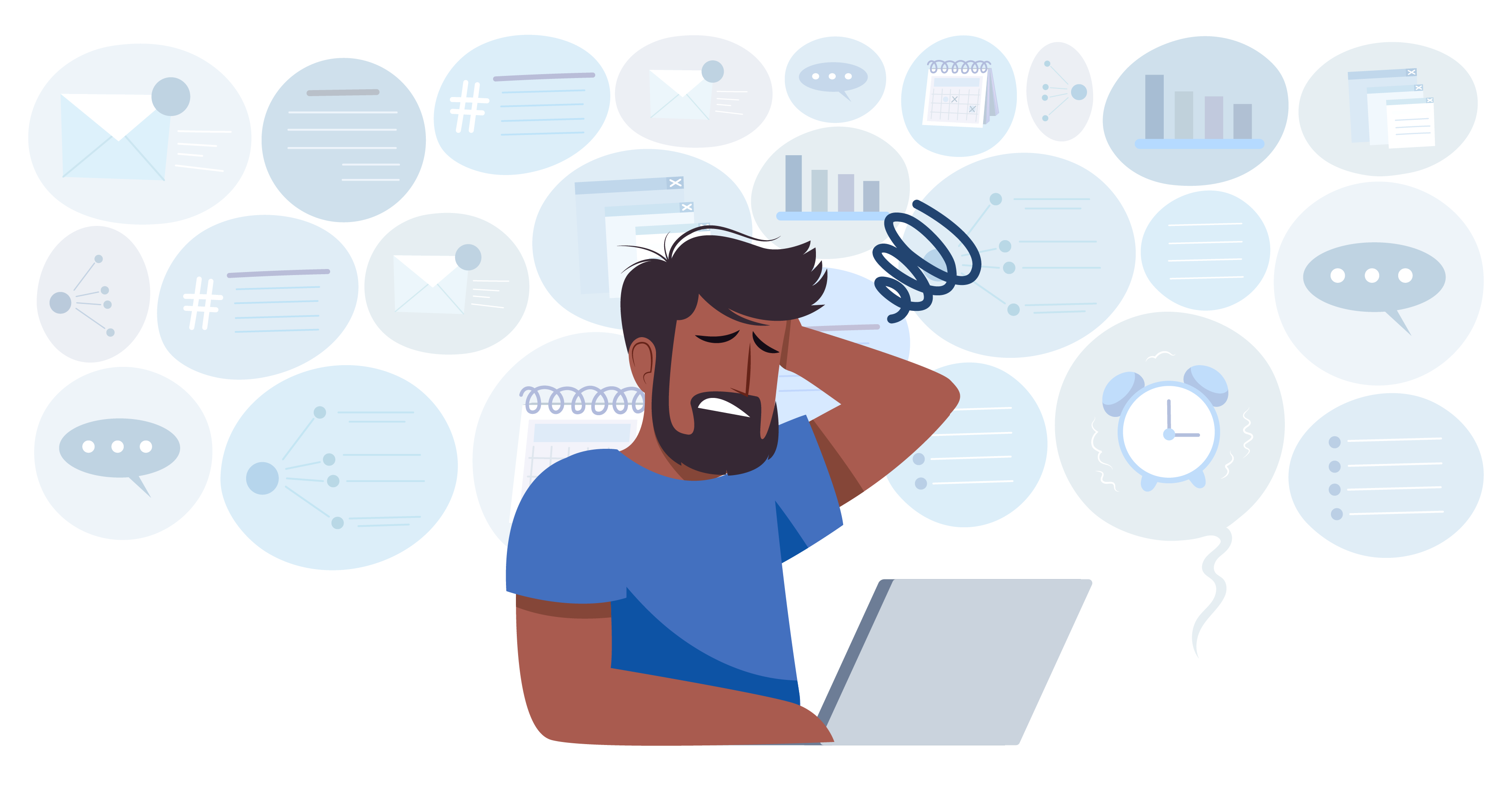
Addressing the aforementioned barriers requires firms to invest in robust systems for organizing, standardizing, and cleaning data. To achieve this, automation is key, as manual data preparation is time-consuming and prone to errors. AEC firms need to invest in tools and strategies that focus on the following:
- Data Ingestion: Systems capable of importing data from various sources (e.g., internal servers, cloud storage, project management platforms) and different formats (structured and unstructured). AI-powered systems can assist in automatically extracting relevant information from documents and files.
- Data Understanding: Tools and other AI-powered algorithms to comprehend the content and context of documents. For example, AI systems that recognize proposals, patterns in architectural drawings, construction schedules, or material specifications.
- Automated Organization: Intelligent classification systems that can categorize data based on its content and relevance. This involves the creation of automated workflows that assign metadata, tags, and file categories based on document type, project stage, or other key factors.
- Data Cleaning: Automated systems that can identify and remove duplicate data, correct errors, and standardize formats. These systems apply predefined rules and machine learning algorithms to detect anomalies or inconsistencies, ensuring that only relevant, high-quality data is fed into AI models.
- Data Integration: Beyond just organizing data, AEC firms need to ensure seamless integration of new and legacy systems. AI-driven platforms should be able to access and analyze data from multiple sources, whether from historical BIM models, project management software and past project material.
By focusing on these areas, AEC firms can streamline their data management processes, reducing the manual effort needed to prepare data and allowing AI systems to extract meaningful insights faster and more efficiently. Platforms, like Workorb AI, that integrate AI capabilities with data management will provide firms with a competitive advantage, allowing them to tap into the potential of their existing data and accelerate innovation.
Conclusion


The adoption of AI in the AEC industry holds immense promise for improving project efficiency, reducing errors, and driving innovation. However, the journey to fully realizing these benefits is fraught with challenges, particularly around data management and cultural resistance. AEC firms must focus on automating data ingestion, organization, and cleaning processes, while also addressing the organizational barriers that hinder AI adoption.
By recognizing these challenges and making targeted investments in AI-powered platforms and data management solutions, AEC firms can unlock the full potential of their historical and operational data. The focus on automated data organization and cleaning represents a critical first step, laying the groundwork for advanced AI applications, such as predictive analytics, generative design, and autonomous project management. Embracing AI today will enable AEC firms to remain competitive, agile, and innovative in an increasingly digitized and data-driven industry. Learn more at workorb.ai.